Loading... # 机器学习3 ## 神经网络 ### 非线性假设 使用非线性的多项式能够帮助我们构建更好的分类模型,但是特征太多会导致计算量大增 普通的逻辑回归无法有效处理太多特征,这时需要神经网络 ### 神经元和大脑 尝试模仿人类大脑,计算量大(当前计算机足够支持) 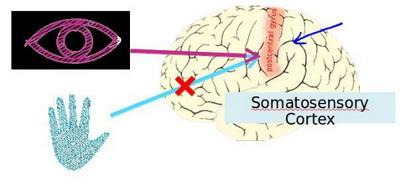 神经网络原因:现代机器学习应用 ### 模型展示 多个输入/树突(dendrite),一个输出/轴突(axon) 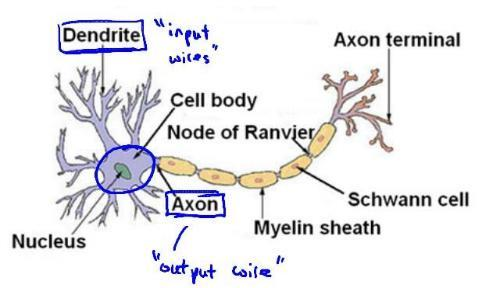 神经元(学习模型/激活单元):多输入一输出,x0称为偏执单元 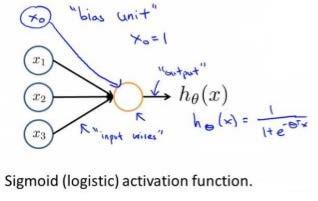 在神经网络中把θ向量称为权重 神经网络图如下: 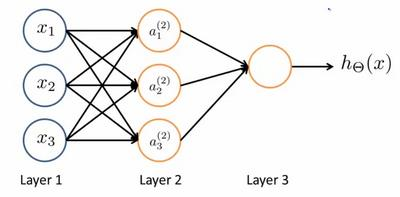 分别为输入层、隐藏层、输出层:隐藏层用来计算复杂特征 为每一层增加一个偏差单位(bias unit) 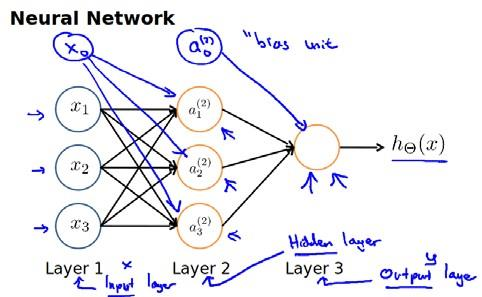 此时$a_i^{(j)}$代表第j层的第i个激活单元,$\theta^{(j)}$代表从第j层映射到第j+1层的权重矩阵;则上图的激活单元和输出分别表达为 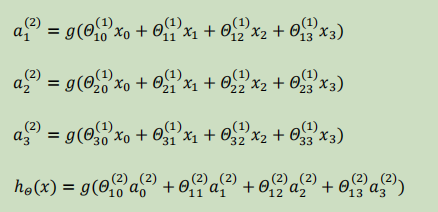 $\theta^{(j)}$为$s_{j+1}\times(s_j+1)$维度,即第j+1层单元数乘以第j层单元数+1【偏差单元】的和 ### 例子与直观理解 **逻辑与**(and) 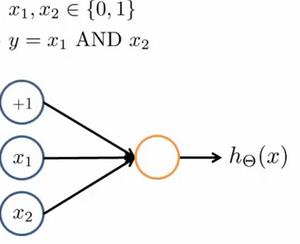 eg:θ0=-30, θ1=20, θ2=20 hθ(x) = g(−30 + 20x1 +20x2) **逻辑或**(or) 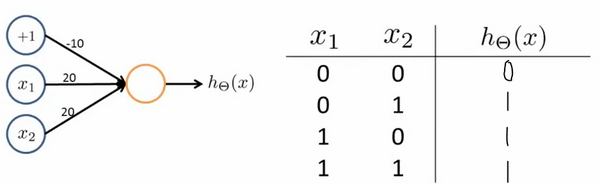 **逻辑非**(not) 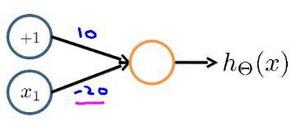 **异或**(xor) XNOR = (x1 AND x2) OR((NOT x1)AND(NOT x2)) 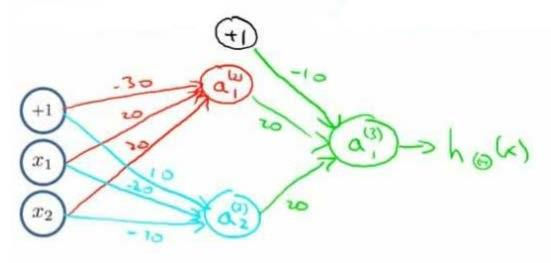 ### 多元分类 eg:每个向量中为1的为一类 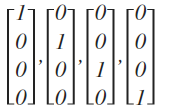 ### 代价函数 最后修改:2022 年 08 月 19 日 © 允许规范转载 打赏 赞赏作者 支付宝微信 赞 0 如果觉得我的文章对你有用,请随意赞赏