Loading... # pytorch tensor处理 ## permute 类似于np.transpose,将tensor的维度换位,只能Tensor.permute() eg: 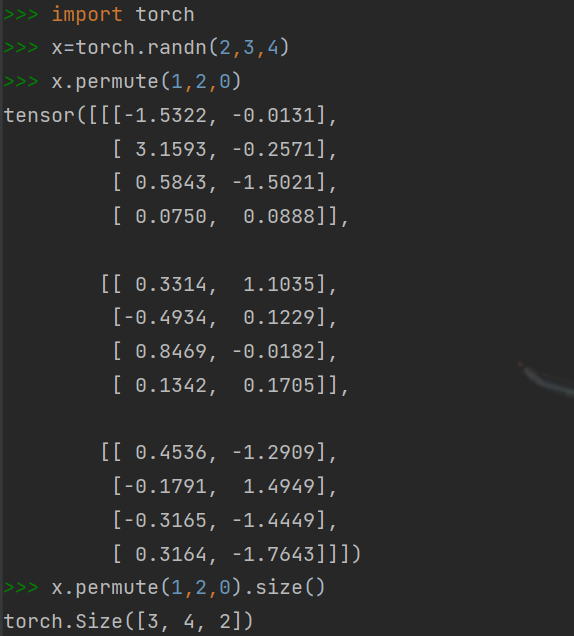 作为对比,torch.transpose()只能操作2D矩阵的转置,即一次作用于tensor的两个维度 ## contiguous pytorch中Tensor是按行优先一维展开的,但是某些Tensor操作后比如transpose、permute等,与原Tensor是共享内存中的数据,不会改变底层数组的存储,语义上相邻,内存不相邻,即不连续了,直接使用view会报错,需要使用contiguous 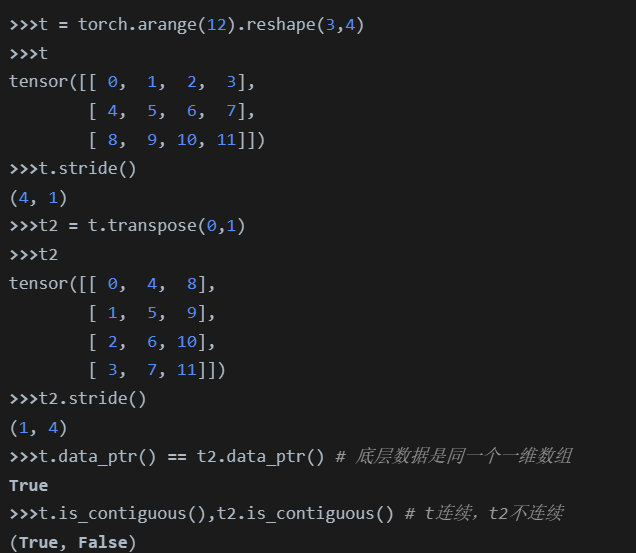 t2和t引用的是同一份底层数据[ 0, 1, 2, 3, 4, 5, 6, 7, 8, 9, 10, 11] 使用contiguous相当于重新开辟了一块内存,并按照新的Tensor按行一维展开,改变了底层数据 所以可以考虑用torch.reshape()替代tensor.contiguous().view(),省去了调用麻烦 ## view 相当于resize(),根据输入形状重新排列tensor ## expand 返回tensor的一个新视图,单个维度扩大为更大的尺寸(复制) eg: 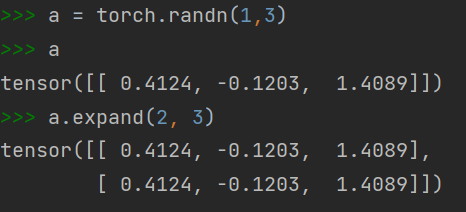 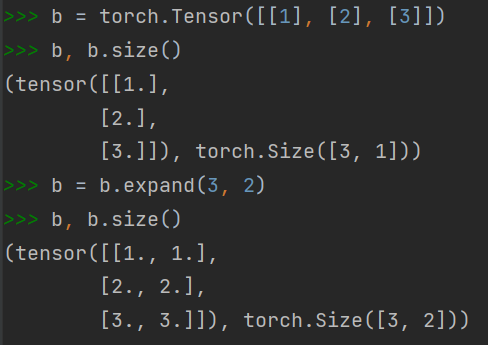 ## torch.empty_like(x) 返回和x形状相同的使用未初始化值填充的tensor ## torch.bernoulli() torch.bernoulli(input)表示从伯努利分布中提取二进制随机数0或1,输入张量应为包含用于绘制二进制随机数的概率的张量,因此输入中的所有值都必须在(0,1)范围内 最后修改:2022 年 10 月 14 日 © 允许规范转载 打赏 赞赏作者 支付宝微信 赞 0 如果觉得我的文章对你有用,请随意赞赏